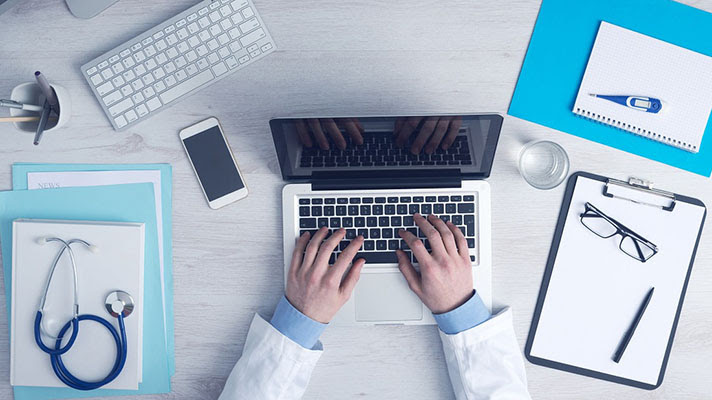
5 Things to Know About the Clinical Analytics Data Management Challenge
Achieving Data Integrity and Accuracy Through a Data First Approach
1. The EHR revolution has created immense promise for improved patient outcomes and reduced costs but many problems exist
Every year new academic reviews reveal a mixed bag of results from the dramatic “digitization” of medical practices that has occurred over the last decade. Some have noted that during the first two years of even the most ‘successful’ EHR implementation, clinician productivity dropped as much as 30%, and correlated with no appreciable increase in quality and safety.
Realigned Incentives, cloud-based technology tools, advanced software tools, and novel delivery models are all necessary for successful care transformation, but are still alone insufficient to reach quality and safety goals. Health systems must apply a comprehensive approach to data accuracy and relevance in order to achieve the clinical transformation and re-engineering necessary for truly effective population health management. To deny this reality, if only tacitly through actions (or inaction), is to subscribe to clinical analytics alchemy—that is, inputting leaden data and expecting a golden output.
2. Your data lake is probably a data swamp
Your healthcare enterprise may have the tools and data you need, but it’s likely that your data lake is more like a data swamp. Data mart proliferation from systems like EHR, ERP, population health platforms, BI and others can leave you with an inflexible model that serves neither your clinical nor IT needs.
Data integrity problems persist as an ongoing issue for healthcare providers. What data integrity essentially means is that the data is accurate and hasn’t been wrongfully altered in any way. Inaccurate data has the potential to become major health risks for patients and a huge liability for providers, leading to such issues as fraud, abuse, data loss, incorrect or incomplete care.
Find out more about extracting real benefit from your EHR data and Symphhony’s Applied Clinical Analytics data management model.
Applied Clinical Analytics - A Data First Approach
Read more about Symphony’s “data-first,” vendor agnostic approach to clinical analytics
3. Clinical Analytics software solutions are only as useful as the data that goes into them
Existing clinical analytics software products, whether off the shelf or specifically configured towards the management of a set of business processes, can do the job that they are intended to do. While not all solutions are created equal, various categories of tools can be readily assembled to address the population health management life cycle.
Even if a health system is able to implement sophisticated population health and supporting clinical analytics software tools, they will ultimately be limited by the data that they can wholly access. If a healthcare organization is generating chaotic, incomplete, or clinically unavailable data, then no amount of technology or workflow engineering will save you from the quality and cost consequences that are likely to result.
4. Smart data not big data rules the day
Current data management challenges are further complicated by the impending massive influx of data from non-core transactional systems, ancillary healthcare data, patient-generated biometrics from non-clinical sources, and rapidly emerging genomics and molecular medicine sources. Where does it all belong? Many in our industry are heralding this growth in both volume and velocity as an opportunity for big data methods and technologies to deliver value as it has in many other industries and data science endeavors. A caveat is necessary here. Although big data technologies and tools are growing more accessible and ubiquitous, the real value to the clinicians who are delivering frontline care to patients is not dependent on the volume of data available about the patient in question but rather upon the accuracy of the data.
Smart data comes into existence when it is generated and delivered through a clearly delineated and traceable data value chain. It comes from a discrete place in a transactional system.
5. Applied Clinical Analytics – A data first approach
Good discipline, governance, and the automation of data processes are vastly under-appreciated for their criticality in delivering on the analytics promise.
Applied Clinical Analytics – A data first approach, brings actionable clinical intelligence to the point of care. This increases clinician efficiency without sacrificing any of the core IT goals related to security and privacy.
ACA’s elegantly simple data solutions, when brought to the point of care through the chart, or even through something as rudimentary as a report, can unlock the power from the data warehouse and accentuate any existing population health and clinical analytics programs.
The power of Applied Clinical Analytics lies in a simple but powerful concept: the importance of focusing on the accuracy and availability of the underlying data, first and foremost. The importance of the right data stewardship, quality assurance, and metadata management as a solid foundation cannot be underestimated.